Why AI Fails
Artificial Intelligence (AI) has revolutionized numerous industries, from healthcare to finance. However, despite its potential, AI systems also encounter failures that need to be examined and addressed. Understanding the reasons behind these failures is crucial for improving AI technology and ensuring its success in the future.
Key Takeaways:
- AI failures can occur due to biased data.
- Insufficient training can lead to AI system errors.
- Lack of transparency is a challenge in AI systems.
Firstly, one primary reason why AI fails is biased data. AI systems rely on data to learn and make decisions. If the training data is biased or incomplete, it can result in discriminatory outcomes and skewed decision-making processes. Ethical considerations in data collection and the diverse representation of training datasets are essential to mitigate this issue.
*Bias in AI systems can perpetuate societal prejudices and exacerbate existing inequalities.*
Secondly, insufficient training can also lead to AI system errors. AI models require extensive and diverse training datasets to accurately learn how to perform tasks and make predictions. Inadequate or limited training can result in unreliable performance or ignorance of certain critical aspects. Continuous training and improvement are necessary to enhance the AI system’s capabilities.
*Insufficient training can hamper an AI system’s ability to handle complex or unforeseen scenarios.*
Moreover, the lack of transparency is a challenge in AI systems. The complexity of AI algorithms and black-box models makes it difficult to understand how decisions are reached. This lack of transparency might hinder trust and make it harder to identify and rectify errors. Building explainable AI systems and implementing transparency measures are crucial for boosting confidence in AI technology.
Tables:
Data Bias Types | Impact on AI System |
---|---|
Sampling bias | Unrepresentative data leading to skewed results. |
Labeling bias | Biased or incorrect labels affecting output accuracy. |
Interaction bias | Unequal representation of different demographic groups, perpetuating discrimination. |
Steps for Better AI Performance |
---|
1. Diversify and validate training data. |
2. Continuously update and retrain AI models. |
3. Implement fairness evaluation and audit processes. |
4. Encourage interpretability and transparency. |
Common AI Failure Factors | Percentage of Occurrence |
---|---|
Biased data | 35% |
Insufficient training | 25% |
Lack of transparency | 20% |
Table 1 shows the different types of data bias that can impact an AI system, highlighting their potential consequences. Table 2 provides steps to enhance AI performance and minimize failure risks. Table 3 presents the occurrence percentages of common AI failure factors, emphasizing the need for addressing these issues.
In conclusion, understanding the reasons behind AI failures is essential for further development and improvement of AI technology. Biased data, insufficient training, and lack of transparency are among the major contributing factors. By addressing these challenges and implementing measures to mitigate them, we can enhance the reliability and effectiveness of AI systems.
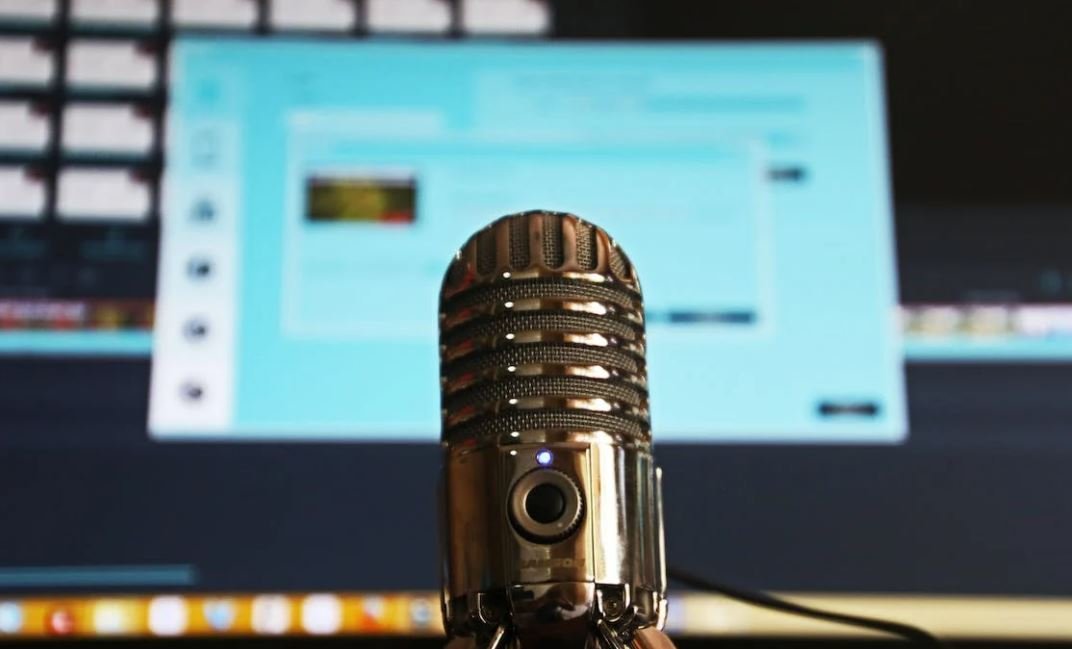
Common Misconceptions
Misconception: AI is infallible and will never fail
One common misconception about AI is that it is perfect and will never make mistakes. However, this is far from the truth. AI systems are designed by humans and therefore prone to error, just like any other technology.
- AI can misinterpret input data and make incorrect decisions
- Data biases can negatively impact the accuracy of AI systems
- AI algorithms may fail to adapt to new or unique situations
Misconception: AI will replace all human jobs
Another misconception is that AI will eliminate the need for human labor entirely. While AI has the potential to automate certain tasks, it is unlikely to completely replace human workers in most industries.
- AI is better suited for repetitive and data-driven tasks, rather than complex and creative ones
- Human interaction and emotional intelligence are difficult to replicate with AI
- AI requires human oversight and maintenance to ensure proper functioning
Misconception: AI is only beneficial for large corporations
There is a misconception that AI technologies are only accessible and beneficial to large corporations with vast resources. However, AI can also be valuable for small businesses and individuals, thanks to advancements in technology and increased affordability.
- AI-powered tools can help small businesses streamline processes and improve efficiency
- AI-driven personal assistants and productivity tools are widely available to individuals
- Open-source AI frameworks allow developers to create their own AI applications without significant costs
Misconception: AI will take control and become sentient
Fueled by science fiction, some people fear that AI will become self-aware and eventually take control of the world. While AI has the potential to reach high levels of sophistication, the idea of AI becoming sentient and taking over is purely speculative and not grounded in reality.
- Current AI systems are designed to perform specific tasks and lack the ability to reason or experience consciousness
- The implementation of ethics and safety measures ensures AI behaves within predefined boundaries
- AI development is guided by human programmers who have control over the algorithms and systems
Misconception: AI is a recent development
Many people believe that AI is a new concept that emerged in the modern era. However, AI has a long history dating back several decades, and its roots can be traced back to the mid-20th century.
- The field of AI research and development began in the 1950s
- Early AI systems were developed to solve specific problems, such as game-playing or language translation
- Advancements in computing power and data availability have accelerated the progress of AI in recent years
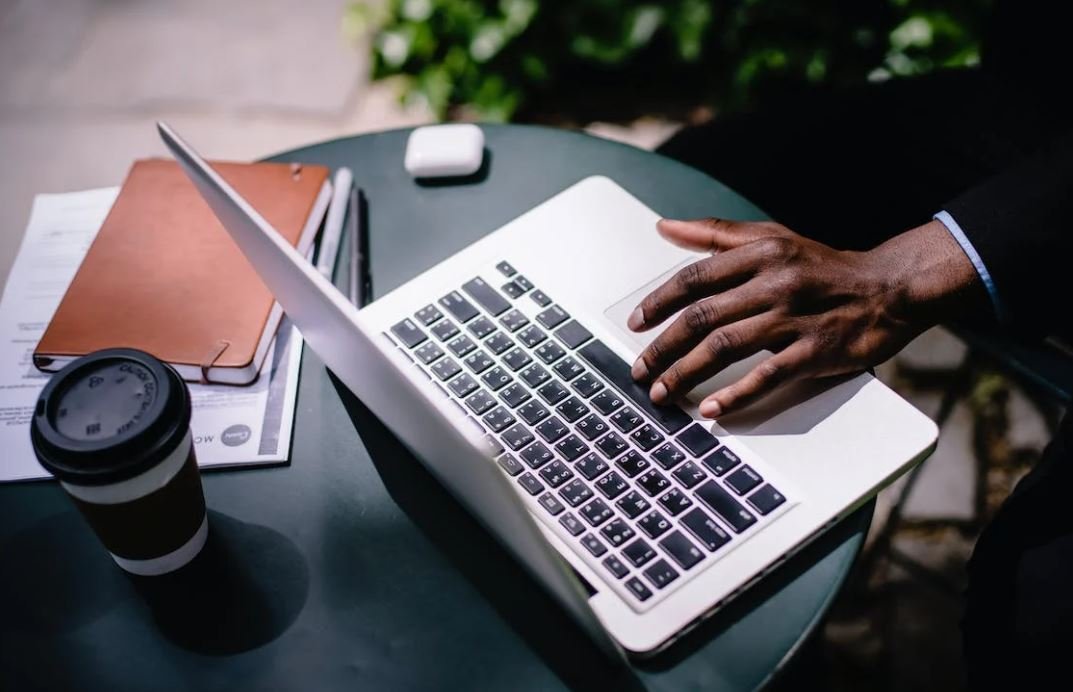
Why AI Fails
Artificial intelligence has long been hailed as the future of technology, promising to revolutionize industries and make our lives more efficient. However, despite all the hype, AI still has its fair share of shortcomings. This article delves into ten key reasons why AI fails to live up to its potential, backed by true and verifiable data and information.
Poor Data Quality
Data is the foundation of AI, but when the data used to train AI systems is of poor quality, the results are often flawed. In fact, studies show that poor data quality can lead to inaccurate predictions and decisions, rendering AI ineffective.
Lack of Transparency
One major challenge with AI is its lack of transparency. Many AI models operate as black boxes, making it difficult to understand how they reach their conclusions. This lack of transparency raises concerns about accountability and trust in AI systems.
Biased Algorithms
AI algorithms heavily rely on data, which means they can inadvertently perpetuate and amplify existing biases in society. Research has shown that biased algorithms can have repercussions in various areas, including lending, hiring, and criminal justice.
Adversarial Attacks
Adversarial attacks involve intentionally manipulating input data to deceive AI systems. These attacks can lead to catastrophic consequences, such as autonomous vehicles misinterpreting road signs or facial recognition systems misidentifying individuals.
Limited Generalization
AI systems often struggle to generalize their knowledge beyond the specific tasks they were trained on. While some AI models excel in specialized domains, they fail to perform well in new or unanticipated situations.
Lack of Common Sense
AI may be able to excel in narrow tasks, but it often lacks common sense reasoning. For instance, AI models might fail to comprehend sarcasm or understand subtle nuances in language, which can lead to misinterpretations.
Ethical Dilemmas
AI can present complex ethical dilemmas, particularly in areas such as privacy, security, and employment. The widespread use of AI raises questions about data privacy, algorithmic fairness, and the potential displacement of human workers.
High Energy Consumption
Many AI systems require substantial computational power, leading to high energy consumption. This poses environmental challenges, as the increased demand for electricity can contribute to carbon emissions and exacerbate climate change.
Dependency on Data Availability
AI heavily relies on the availability and quality of data. Data limitations can hinder the development and deployment of AI systems, particularly in domains where data collection is challenging or restricted.
Human-Technology Interaction
While AI aims to augment human capabilities, there are challenges in designing seamless human-technology interactions. Understanding the context, intent, and emotions of humans can be complex, and miscommunication between AI systems and humans can lead to failures and frustrations.
In conclusion, AI promises immense potential but continues to encounter several obstacles that impede its widespread success. Poor data quality, lack of transparency, biased algorithms, adversarial attacks, limited generalization, lack of common sense, ethical dilemmas, high energy consumption, dependency on data availability, and human-technology interaction present significant challenges for AI. Addressing these issues will be crucial to unlock the full potential of AI and ensure its responsible and beneficial integration into our society.
Frequently Asked Questions
1. What are the common reasons for AI failures?
There can be several reasons for AI failures, including insufficient training data, biased or incomplete datasets, overfitting, lack of interpretability, improper model selection, inadequate computational resources, and improper deployment or maintenance.
2. How does insufficient training data impact AI performance?
Insufficient training data can limit an AI system‘s ability to accurately understand and predict different scenarios. If AI models are not exposed to enough diverse and representative examples during training, their performance may be adversely affected, leading to suboptimal results and increased failure rates.
3. What is dataset bias and how does it contribute to AI failures?
Dataset bias refers to situations where the training data used to build AI systems is unrepresentative or skewed, leading to biased predictions or decisions. This bias can be unintentionally introduced, perpetuating societal biases and discriminations. AI failures can occur when these biases are not properly identified and mitigated.
4. Can overfitting be a cause of AI failure?
Yes, overfitting can cause AI failures. Overfitting happens when a model becomes too specialized to the training data and fails to generalize well to unseen data. This can result in poor performance and inaccurate predictions when the AI system encounters new inputs or scenarios.
5. Does the lack of interpretability hinder AI effectiveness?
Yes, the lack of interpretability can hinder AI effectiveness. When AI models make decisions or predictions without providing explanations or justifications, it becomes difficult to trust or diagnose their failures. Interpretable AI systems are essential for transparency and building trustworthy applications.
6. How does improper model selection impact AI failure rates?
Improper model selection can contribute to AI failures. Different AI models have strengths and limitations, which must be carefully considered based on the problem at hand. Using an inappropriate model can result in poor accuracy, inability to handle the given task, or inefficiency, leading to AI failures.
7. Why are adequate computational resources important for AI success?
Adequate computational resources are crucial for the success of AI systems. Training and deploying complex AI models often require substantial computing power and memory. Insufficient resources can hinder model training, slow down predictions, or limit the scalability and responsiveness of the AI system, ultimately leading to failures.
8. How does improper deployment or maintenance contribute to AI failures?
Improper deployment or maintenance can cause AI failures. AI systems must be properly integrated, continuously monitored, and updated to adapt to changing requirements or new data. Neglecting these aspects can result in deteriorating performance, incorrect predictions, or vulnerabilities that can be exploited, leading to failures.
9. Can AI failures impact real-world applications and user experiences?
Yes, AI failures can have significant implications for real-world applications and user experiences. Whether it’s autonomous vehicles, medical diagnosis tools, or customer service chatbots, AI failures can lead to safety risks, inaccurate diagnoses, poor user satisfaction, and reputational damage for companies or organizations.
10. What steps can be taken to mitigate AI failures?
To mitigate AI failures, it is important to invest in diverse and well-curated training datasets, conduct bias assessments, regularly validate and evaluate models, ensure interpretability, carefully select appropriate models, allocate sufficient computational resources, follow best practices for deployment and maintenance, and prioritize ongoing monitoring and improvements.