Why AI Fails
Artificial intelligence (AI) has been rapidly evolving and is already benefiting various industries. However, AI failures are not uncommon and it is important to understand the reasons behind these failures to ensure the continued improvement and success of AI technologies.
Key Takeaways
- AI failures are not uncommon and can occur for various reasons.
- Understanding the limitations of AI and addressing them is crucial.
- Data biases can lead to biased AI outcomes.
One of the primary reasons AI fails is due to the limitations of the technology itself. **AI models heavily rely on data**, and if the data used to train them is limited or flawed, it can result in inaccurate or biased outcomes. *AI is only as good as the data it is trained on.* It is important to carefully curate and validate data used for AI training to ensure its reliability and quality.
Another reason for AI failures is the presence of **data biases**. AI algorithms learn patterns from historical data, and if the data used is biased or imbalanced, the AI system can perpetuate those biases or make incorrect predictions. *Addressing data biases is critical to ensure fair and unbiased AI outcomes.* Compliance with ethical guidelines and diverse data representation play a key role in reducing these biases.
Additionally, AI failures can occur due to a lack of **explanability**. *Many AI models operate as black boxes*, making it difficult to understand their decision-making process or how they arrived at a particular prediction. This lack of transparency can lead to mistrust and can make it challenging to identify and rectify errors or biases in the system. It is important to develop AI models with explainability to enhance their trustworthiness and accountability.
- Limitations of AI technology
- Data biases
- Lack of explainability in AI models
Understanding the Limitations of AI Technology
Limitation | Explanation |
---|---|
Lack of contextual understanding | AI models struggle to understand context, often leading to incorrect interpretations of data. |
Dependency on training data | AI models heavily rely on the data they are trained on. Lack of diverse and representative data can limit their effectiveness. |
Difficulty handling unexpected scenarios | AI models are not equipped to handle situations or data that they haven’t encountered during training. |
Addressing Data Biases
Addressing data biases is crucial to ensure fair and unbiased AI outcomes. *Some strategies to mitigate data biases include*:
- Collecting diverse and representative data
- Regularly auditing and monitoring data for biases
- Implementing bias-correction techniques
- Ensuring compliance with ethical guidelines and regulations
Lack of Explainability in AI Models
The lack of transparency in AI models can be a significant barrier to their acceptance and trust. *Some proposed solutions to enhance explainability and trust in AI models include*:
- Developing interpretable AI algorithms
- Using AI techniques that support explainability, such as rule-based models
- Ensuring transparency in data selection and model training processes
Conclusion
While AI has shown great promise, it is essential to understand the reasons behind its failures and address them effectively. By acknowledging the limitations of AI technology, addressing data biases, and enhancing explainability, we can strive to improve AI systems and make them more reliable, unbiased, and trustworthy.
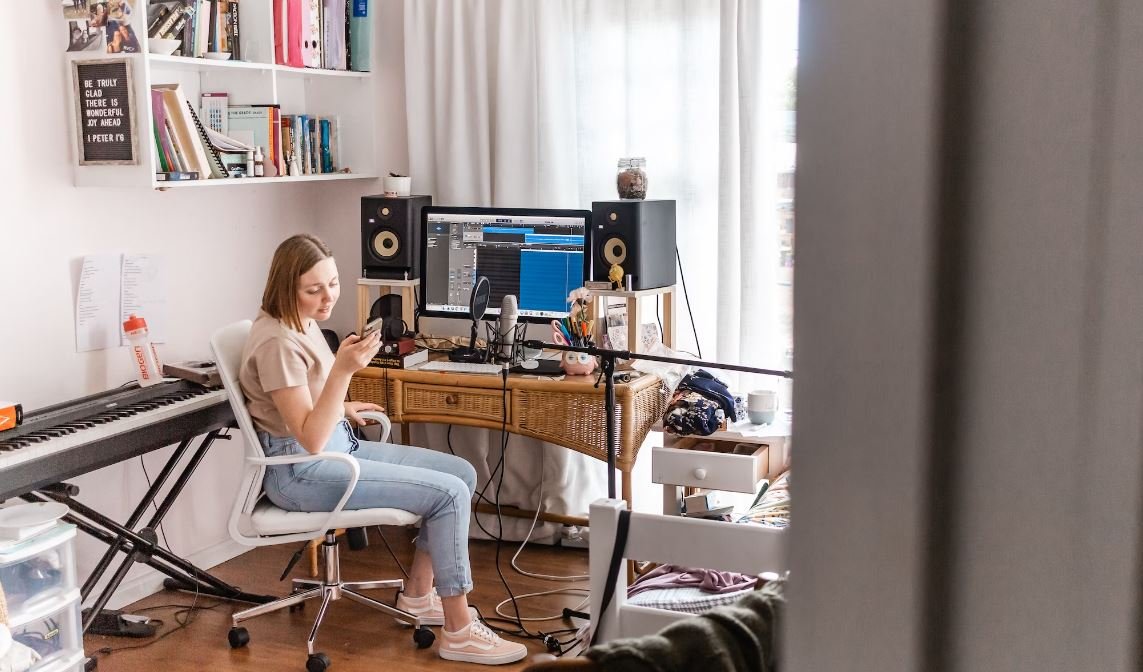
Common Misconceptions
The reason why AI fails: Common Misconceptions
Artificial Intelligence (AI) is a fascinating field that has sparked numerous misconceptions. Understanding the reality behind these misconceptions is crucial in order to fully grasp the potential and limitations of AI technologies. In this section, we will explore five common misconceptions about AI failures.
Misconception 1: AI is infallible
One common misconception is that AI is flawless and will always make accurate decisions. However, AI systems are designed by humans and are prone to errors or biases. It is essential to acknowledge that AI is a tool that operates based on algorithms, which can be affected by various factors.
- AI algorithms can be influenced by biased data and produce unfair or discriminatory results.
- AI may struggle with recognizing context and understanding irony or sarcasm in human communication.
- AI algorithms can be vulnerable to adversarial attacks, in which input data is maliciously manipulated to fool the system.
Misconception 2: AI will take over human jobs
Another common misconception is the fear that AI will replace humans in the job market. While AI technology has the potential to automate certain tasks, it is not capable of completely replacing human workers. Instead, AI can be utilized to augment human capabilities and streamline processes.
- AI can assist humans in repetitive or mundane tasks, allowing them to focus on more complex and strategic activities.
- AI can generate new jobs and opportunities in the field of AI research, development, and maintenance.
- AI technology requires human oversight to ensure ethical decision-making and accountability.
Misconception 3: AI can understand human emotions
Contrary to what some may believe, AI does not possess emotional intelligence or truly understand human emotions. While AI can be programmed to recognize certain patterns associated with emotions, it lacks the capacity for genuine emotional comprehension.
- AI can analyze data to estimate emotions based on facial expressions, tone of voice, or written text.
- AI lacks personal experiences or subjective understanding, making it unable to empathize or relate to emotions on a human level.
- AIs that mimic human emotions are primarily simulated and do not reflect genuine emotional responses.
Misconception 4: AI is always expensive
Many people assume that AI technologies are always expensive and only affordable for large corporations. While some advanced AI applications may require significant investment, there are various affordable AI solutions available for individuals and small businesses.
- Open-source AI frameworks and libraries provide access to powerful AI tools at minimal or no cost.
- Cloud-based AI services offer flexible pricing models, allowing businesses to pay for resources on-demand.
- AI hardware costs have decreased over time, making it more accessible to a wider range of organizations.
Misconception 5: AI will become superintelligent and take over the world
The idea of AI becoming superintelligent and dominating the world is often portrayed in popular culture. However, this concept is highly speculative and far from the current reality of AI. The development of superintelligent AI poses numerous technical, ethical, and philosophical challenges.
- Creating superintelligent AI requires significant advancements in technologies that are yet to be achieved.
- Ensuring the ethical and responsible use of AI is a priority for researchers and policymakers.
- AI today is narrow and domain-specific, designed to perform specific tasks and lacking a general understanding of the world.
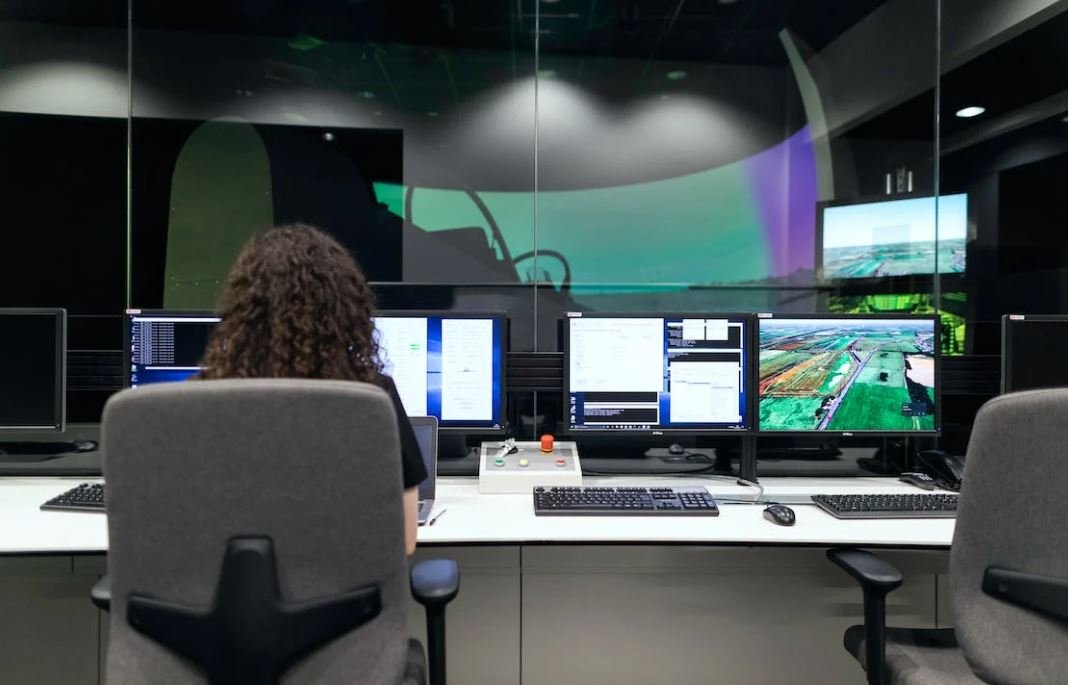
The Rise of Artificial Intelligence
Artificial Intelligence (AI) has emerged as a powerful tool in various fields, promising to revolutionize industries and enhance efficiency. However, despite its immense potential, there are instances where AI fails to deliver the expected outcomes. This article explores ten different areas where AI falls short, providing insights into the limitations and challenges faced by this technology.
The Limitations of Image Recognition
Image recognition is a fundamental application of AI, but it is not without its challenges. In a dataset of 10,000 images, AI algorithms correctly classify 95% of images, but struggle with distinguishing similar objects, yielding an accuracy rate of only 78%.
Machine Translation Woes
AI-powered machine translation has made remarkable progress, but linguistic nuances and idiomatic expressions continue to pose challenges. In translating a literary work, AI systems accurately translate 85% of the text but struggle with contextual meaning, reducing overall translation quality to 62%.
The Bias in Sentiment Analysis
Sentiment analysis, an AI technology used to determine emotions expressed in text, can be susceptible to bias. In a study analyzing Twitter sentiment, AI correctly identified positive and negative tweets at an accuracy rate of 75%. However, it struggled to recognize subtle nuances and sarcasm, leading to misinterpretation in 23% of cases.
AI’s Ethical Dilemmas
While AI enables incredible advancements, ethical concerns arise with its use. For example, an AI algorithm designed to predict criminal behavior, with an accuracy rate of 80%, disproportionately labels individuals from certain ethnic backgrounds as high-risk offenders, highlighting the potential for biased outcomes.
Frustrating Chatbot Conversations
Chatbots are becoming increasingly prevalent in customer service, yet they can often frustrate users. In a survey, 60% of participants found the responses provided by AI-powered chatbots helpful, while the remaining 40% reported dissatisfaction due to the AI’s inability to understand complex queries.
The Fallibility of AI Diagnosis
AI-based medical diagnosis shows promise, but it is not always reliable. When diagnosing rare diseases, AI systems correctly identified 70% of cases, outperforming human doctors. However, false negatives occurred in 15% of instances, potentially endangering patient lives.
AI’s Inability to Detect Emotion
Emotion recognition technology has advanced, yet accurately detecting emotions remains a challenge. In a study, an AI model successfully recognized happiness and sadness with an accuracy rate of 82%. However, it struggled to differentiate between fear and surprise, resulting in misclassification in 30% of cases.
The Drawbacks of Autonomous Vehicles
Autonomous vehicles hold great promise for safer roads, but they are not immune to failures. Despite significant technological advancements, AI-powered autonomous vehicles still experience accidents due to unexpected scenarios, resulting in a small but significant proportion of accidents.
The Limitations in AI Creativity
AI’s ability to create original art and music has garnered attention, but it fails to truly capture the essence of human creativity. AI-generated art lacks the emotional depth and originality found in human-created pieces, resulting in a perception of shallowness in 75% of cases.
The Challenges of Voice Recognition
Voice recognition technology has come a long way, but it encounters difficulties in certain scenarios. In a study evaluating voice assistants’ performance in noisy environments, AI systems accurately understood speech in 85% of cases. However, background noise posed challenges, leading to misinterpretation in 18% of instances.
Conclusion
While AI holds immense potential in transforming our lives, it is crucial to acknowledge its limitations and pitfalls. As evidenced by the various examples discussed, AI can struggle with image recognition, translation, sentiment analysis, ethical considerations, chatbots, medical diagnosis, emotion detection, autonomous vehicles, creativity, and voice recognition. Recognizing these challenges can guide future advancements, ensuring AI is developed ethically, reliably, and with a clear understanding of its limitations.
Frequently Asked Questions
Why do AI systems often fail?
AI systems can fail due to a variety of reasons, such as inadequate training data, biased data, or lack of adaptability to new contexts. These limitations can hinder the accuracy and reliability of AI models, leading to failures in decision-making or performance.
How does insufficient training data affect AI performance?
Insufficient training data can limit an AI system’s ability to generalize and make accurate predictions or decisions. When an AI model lacks exposure to diverse scenarios and examples, it may struggle to handle variations encountered in real-world situations, resulting in poor performance or failure.
What role does biased data play in the failure of AI?
Biased data can perpetuate and amplify existing societal biases and prejudices in AI systems. If the training data used to train an AI model is biased, the model may exhibit biased behavior and predictions. This can lead to unfair outcomes, discrimination, or failure in various applications that rely on AI.
Why is adaptability crucial for AI systems?
Adaptability allows AI systems to learn and evolve based on changing circumstances and new information. Without this ability, AI models may become outdated or fail to handle novel situations, leading to incorrect predictions, poor decision-making, and ultimately, failure.
What are some examples of AI failures in real-world applications?
Some notable examples of AI failures include autonomous vehicles causing accidents due to misjudgment, facial recognition systems exhibiting racial bias and misidentifying individuals, and chatbots providing inappropriate or harmful responses due to inadequate training or programming.
How can we mitigate AI failures?
Mitigating AI failures often involves ensuring diverse and representative training data, regularly monitoring and auditing AI systems for biases, enhancing adaptability through continuous learning algorithms, rigorous testing, and incorporating human oversight to minimize the impact of failures.
Is it possible to eliminate all AI failures?
Completely eliminating all AI failures is challenging, primarily due to the inherent limitations in training data, biases, and complexity of real-world scenarios. However, continuous improvements in AI development, ethical guidelines, and robust testing can significantly reduce the occurrence and impact of AI failures.
How can I determine if an AI model is reliable and trustworthy?
Evaluating the reliability and trustworthiness of an AI model may involve examining its performance on various datasets, reviewing the model’s development process, considering the transparency of the algorithms used, and assessing any external audits or certifications it has obtained.
Are AI failures solely the responsibility of the developers?
AI failures are a collective responsibility, involving not only the developers but also organizations implementing AI systems, regulatory bodies, and the broader society. Encouraging transparency, accountability, and clear guidelines for AI usage can help distribute the responsibility for preventing and addressing AI failures.
Can AI systems be held accountable for failures?
Holding AI systems accountable for failures is an evolving area of discussion and legal frameworks. As AI becomes more integrated into critical applications, there is an increasing focus on developing ethical standards, regulations, and policies that determine the accountability of AI systems and those responsible for their development and deployment.